What is “Navi2”?
It is the code-name of the new AMD GPU, the 2nd generation RDNA (Radeon DNA) GPU arch(itecture) that itself replaced “Vega” / last of the GCN arch(itecture). Unlike the original Navi that was a mid-range GPU – this is the very much expected “big Navi” top-end GPU designed to battle nVidia’s finest 3000-series GPUs.
Navi/RDNA arch brought big changes from Vega/GCN and Navi2 has been enhanced and optimised from Navi1 adding more features:
- Ray-Tracing (RT) Cores – similar to nVidia’s Turing/Ampere cards
- Infinity Cache – 128MB
- Smart-Access Memory – PCIe BAR re-sizeing
- 6900XT has Navi21 XT top-end chip with all CUs enabled and speed binned
Unlike Vega1/2 GPUs that perhaps were very much compute focused – Navi1/2 seem to be more gaming focused with the few compute features already introduced: reduced workgroup size matching nVidia (32), increased work-group sizes (1024). It is likely that AMD will launch HBM2 professional cards and hopefully Navi versions with tensor units (TSX) or matrix multiplicators (tuMMA).
See these other articles on GP-GPU performance:
- ExtremeTech
- SiSoftware
Hardware Specifications
We are comparing the top-range Radeon with previous generation cards and competing architectures with a view to upgrading to a top-range high performance design.
GP-GPU Specifications | AMD Radeon 6900XT (Navi2X) | AMD Radeon 5700XT (Navi1) | nVidia 3090 (Ampere) | nVidia 2080TI (Turing) | Comments | |
Arch / Chipset | RDNA2 / Navi 21 XT | RDNA1 / Navi 10 | Ampere / GA102 / SM8.6 | Turing / GT102 / SM7.5 | The 2nd of the Navi cores | |
Cores (CU) / Threads (SP) | 80 / 5,120 [+2x] | 40 / 2,560 | 82 / 10,496 | 68 / 4,352 | Twice as many CU as Navi1 | |
Wave/Warp Size | 32 | 32 | 32 | 32 | Wave size now matches nVidia. | |
Speed (Min-Turbo) (GHz) |
1.825 (2.250) | 1.6 (1.755) | 1.4 (1.78) | 1.35 (1.635) | 40% faster base and 20% turbo than Vega1. | |
Power (TDP) | 300W [+33%] | 225W | 350W | 260W | Power has only increased by 33% | |
ROP / TMU | 128 / 320 [+2x] | 64 / 160 | 112 / 328 | 88 / 272 | 2x increase in ROP/TMU. | |
Ray-Tracing (RT) |
80 | none | 82 | 68 | Navi2 brings 80 RT cores like nVidia. | |
Shared Memory (kB) |
64kB | 64kB | 48kB / 96kB per SM | 48kB / 96kB per SM | No change in shared memory. | |
Constant Memory (GB) |
8GB | 4GB | 64kB dedicated | 64kB dedicated | No dedicated constant memory but large. | |
Global Memory (GB) |
16GB GDDR6 16Gbps 256-bit | 8GB GDDR6 14Gbps 256-bit | 11GB GDDR6X 19.5Gbps 384-bit | 11GB GDDR6 14Gbps 320-bit | Memory is 2x larger but speed gets minor bump. | |
Memory Bandwidth (GB/s) |
512GB/s [+14%] | 448GB/s | 936GB/s | 616GB/s | Bandwidth is 9% just higher. | |
L1 Caches (kB) |
32kB / WG + 128kB/Array | 64kB/Array | 82x 128kB/SM | 68x 96kB/SM | L1 has been doubled (2x) | |
L2 Cache (MB) |
4MB | 4MB | 6MB | 5.5MB | L2 has not changed. | |
Maximum Work-group Size |
1024 / 1024 | 1024 / 1024 | 1024 / 2048 per SM | 1024 / 2048 per SM | AMD has unlocked work-group sizes to 4x. | |
FP64/double ratio |
1/16x | 1/16x | 1/32x | 1/32x | Ratio is 2x nVidia. | |
FP16/half ratio |
2x | 2x | 2x | 2x | Ratios are the same throughput. | |
Price (USD) |
999 [+2x] | 450 | 1,500 | 1,000 | Price is 2x Navi1! |
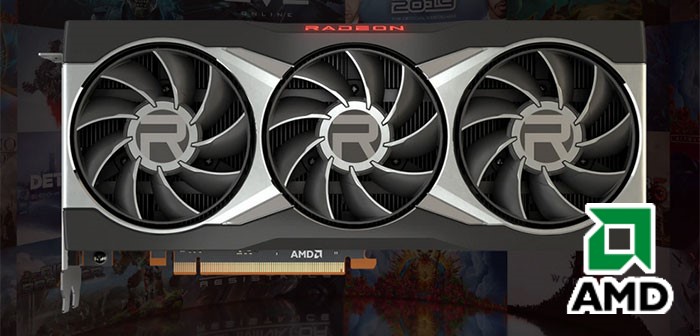
AMD Radeon 6900 XT
Disclaimer
This is an independent article that has not been endorsed nor sponsored by any entity (e.g. AMD). All trademarks acknowledged and used for identification only under fair use.
The article contains only public information (available elsewhere on the Internet) and not provided under NDA nor embargoed. At publication time, the products have not been directly tested by SiSoftware and thus the accuracy of the benchmark scores cannot be verified; however, they appear consistent and do not appear to be false/fake.
Processing Performance
We are testing both OpenCL performance using the latest SDK / libraries / drivers from both AMD and competition.
Results Interpretation: Higher values (GOPS, MB/s, etc.) mean better performance.
Environment: Windows 10 x64, latest AMD and nVidia drivers. Turbo / Boost was enabled on all configurations.
Memory Performance
We are testing both OpenCL performance using the latest SDK / libraries / drivers from AMD and competition.
Results Interpretation: For bandwidth tests (MB/s, etc.) high values mean better performance, for latency tests (ns, etc.) low values mean better performance.
Environment: Windows 10 x64, latest AMD and nVidia. drivers. Turbo / Boost was enabled on all configurations.
SiSoftware Official Ranker Scores
Final Thoughts / Conclusions
Sumary: Decent in compute but not top except for FP64: 8/10 Recommended
Ever since the release of Navi1 (“little-Navi”) and its revolutionary new architecture AMD fans everywhere have eagerly awaited the release of “big-Navi” that can bring the fight to nVidia. A year or so later – we have Navi2: pretty much twice Navi1 + ray-tracing – tensors/tiles. It is everything that was expected but in some ways perhaps we expected more?
Compute (FP16/FP32) performance generally matches expectations – it is usually over twice (2x+) as fast as Navi1 in most algorithms, though in some cases even 9x faster in image processing – showing its “gamer” credentials. Unfortunately, this is insufficient to beat nVidia’s latest “Ampere” top-end cards that are usually 2x faster still – but at least it does trade blows with previous “Turing” generation.
Compute FP64 performance is much better thanks to lower FP32/64 ratio (1/16x vs. nVidia 1/32x) that allows it to beat nVidia’s cards generally by 2x – thus if you use high-precision algorithms – Navi2 can be the better choice.
Memory-wise, it seems the meager bandwidth improvement over Navi1 is holding Navi2 back – with old Vega’s HBM2 memory sorely missed. However, it is likely AMD will use that in its professional cards to battle nVidia’s Titan cards (that also used to have HBM2 e.g. “Volta”). At least now we have twice (16GB) of memory which can hold much bigger kernels – and is larger than nVidia’s 3080 (12GB) but naturally not as large as the top end 3090 (24GB).
The price (USD 1,000) is also twice the old Navi1 price thus you are getting slightly better performance for your money (as it is generally over 2x faster). TDP/Power has gone up by 33% (300W) but efficiency is naturally much better than Navi1 and should not require a new power supply.
In summary – for compute Navi2 is not the same outright success that it is for gamers; it cannot match or beat current competition but does perform well and perhaps with maturing drivers even better. At last you get a great gamer card which you can use for compute (crypto-mining?) during “free time”.
To see how the “little-brother” Navi2L performs see our AMD Radeon RX 6800 (RDNA2, Navi2L) Review & Benchmarks – GPGPU Performance article.
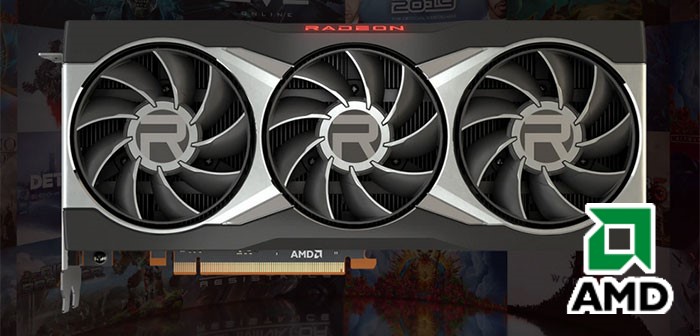
AMD Radeon 6900 XT
Disclaimer
This is an independent article that has not been endorsed nor sponsored by any entity (e.g. AMD). All trademarks acknowledged and used for identification only under fair use.
The article contains only public information (available elsewhere on the Internet) and not provided under NDA nor embargoed. At publication time, the products have not been directly tested by SiSoftware and thus the accuracy of the benchmark scores cannot be verified; however, they appear consistent and do not appear to be false/fake.